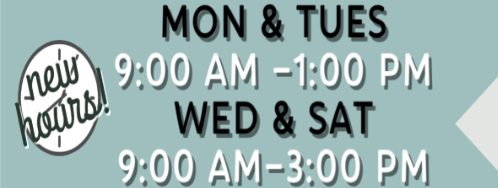
GenLib Crack+ Free 2022 GenLib is the library containing the necessary tools and objects for running algorithms (or parts of them) based on genetic algorithms. For each problem, at least one of the genetic operators should be defined. For the single-objective problems, the ones we support (with example code for each) are: - binary coding - binary tournament - binary tournament with preferential selection of elites - binary tournament with preferential selection of elites with mutation - binary tournament with preferential selection of elites with mutation and crossover - binary tournament with preferential selection of elites with mutation and crossover - binary tournament with one of the following strategies (we're still working on the documentation for the others): - random - rough approximation by a sine curve - rough approximation by a sine curve with mutation - rough approximation by a sine curve with mutation and crossover - rough approximation by a sine curve with mutation, crossover and uniform crossover - rough approximation by a sine curve with mutation, crossover and uniform crossover and preferential selection of elites - rough approximation by a sine curve with mutation, crossover, uniform crossover and preferential selection of elites - random with mutation - rough approximation by a sine curve with mutation and crossover - rough approximation by a sine curve with mutation, crossover, uniform crossover and preferential selection of elites - rough approximation by a sine curve with mutation, crossover, uniform crossover and preferential selection of elites and crossover - rough approximation by a sine curve with mutation, crossover, uniform crossover, preferential selection of elites and crossover The binary coding and binary tournament with mutation are adequate to solve all the problems we've found in real applications, but they're not powerful enough to handle some of the interesting (to us) problems. For these, we provide the following genetic operators: - binary tournament with preferential selection of elites with mutation and crossover - binary tournament with preferential selection of elites with mutation and crossover and crossover - binary tournament with one of the following strategies (we're still working on the documentation for the others): - random - rough approximation by a sine curve - rough approximation by a sine curve with mutation - rough approximation by a sine curve with mutation and crossover - rough approximation by a sine curve with mutation, crossover and uniform crossover - rough approximation by a sine curve with mutation, crossover and uniform crossover and preferential selection of elites - rough approximation by a sine curve with mutation, crossover, uniform crossover and preferential selection of elites and GenLib Crack+ Free Download The library is intended to provide an easy way for users to develop and implement programs to solve various optimization problems. Many of the functions of the library are generic, in that they can be applied to any type of problem (single or multiple objective). The library also provides mechanisms to allow users to implement a variety of methods to solve their problems. In addition, the library is intended to be a flexible tool in that users can add their own functions to support their optimization problems and thereby make use of different methods as they see fit. GenLib can be used as follows: 1) It provides a set of functions and structures which allow a user to implement a variety of optimization functions. 2) It provides an easy mechanism to select the optimization method (algorithms) to be used to solve a specific optimization problem. Some of the main features of GenLib are listed below: 1) Provides routines for solving problems using a variety of optimization methods 2) It provides a set of simple structure and functions which allows a user to easily implement the algorithms. 3) User may use both real and integer variables. 4) Support both single and multiple objective problems. 5) User may create new algorithms by combining other available algorithms. 6) The library provides some mechanism to test user algorithms by using predefined problem sets. 7) It allows a user to override the value of an optimization variables to evaluate the solutions. 8) The library may be used as a stand alone library or together with a third party software. 9) The library does not require any third party software to be installed. 10) It has been tested on a variety of programming platforms. 11) It has been successfully used by a number of research labs as well as by students from across the globe for solving many real world optimization problems. The library itself provides a set of generic routines for implementing optimization functions. The library is based on a well known interface library which enables the users to easily create new functions using the existing library. There are two generic interface classes; one to represent the function itself and the other to represent the basic function that allows a user to easily create a new function by overloading certain basic operations of the interface. There are two generic data structures used by the library to represent a problem, the first to represent the individual points and the second to represent the problem itself. It is worth mentioning that the structure representing the problem is not the same as an individual point. Package Contents: 1) genlib.h – A library header file that contains definition of different type 1a423ce670 GenLib Download The idea of using genetic algorithms has been around for a while, but it was not widely used because of too many different approaches. GenLib is different in the sense that it provides a solid base for writing genetic algorithms. When writing Genetic Algorithm applications the size of the data set may get quite large, which is a problem because Genetic Algorithms usually need to run over all data set to get a good convergence and so good value. One might think of breaking the problem in sub problems and running each one on a small data set and then combining the results, but how to do that without hard coding in each algorithm? GenLib handles this with the key macro. The key macro is described in more detail below. We want to run over all data, so the key macro is a run over the whole data set and then do some calculations. The key macro takes an information structure as an argument, where the information structure contains the algo-definition (i.e. the configuration of the Genetic Algorithm) and other parameters like the capacity to store data. The algo-definition contains the operations to be performed. The capacity to store data is used for inserting and removing records. GenLib supports three types of input structures: Single object Multiple objects Stack In the end, a generic run over a data set is performed by a method (which takes as input the key macro) and the method generates different children, each containing a run over part of the data set. This can then be used to iteratively create different generations of sub-populations, where each sub-population is used to populate a solution variable. Hence a generic run over a data set is achieved by running over the data set (which is stored in the single object input structure), and then generating a list of solutions which is then inserted into the list of solutions. The solution is deleted from the list of solutions when it is replaced by a new solution. Currently the key macro has this implementation (below) : ObjKey.h class ObjKey { public: ObjKey(char* objectName,...); ~ObjKey(); char* getObjectName(); }; ObjKey.cpp ObjKey::ObjKey(char* objectName,...) { // Do code here for constructing object } ObjKey::~ObjKey() { // Do code here for destructing } char* ObjKey What's New In? System Requirements For GenLib: The following system requirements are intended to ensure that Old School RuneScape plays successfully and stably on your device. The recommended specification for mobile devices is a smartphone or tablet with the following specs: 1GHz CPU or faster 512 MB RAM (minimum) Android version 4.4 or higher 4 GB of available storage (minimum) Please note that a recent version of Java is required. This should be installed on your computer before downloading the game. Mobile devices without the specs described here may have problems when playing Old School RuneSc
Related links:
Commentaires